Macrophomina and Prosopis farcta are huge enemies of strawberries and melons. If not using an UAV, an agronomist, particularly field worker, has to go through an entire field and detect issues manually. Drone inspections with further data processing in an analysis software tool like ATLAS by SPH Engineering not only save time to a person in charge of inspections, but also save plant life time. Agro Drone, an Israeli company well associated with agriculture, shares experience of detecting problematic areas with the help of a UAV and AI.
#strawberry
Strawberry fields are prone to various plant diseases during the growing period. One of the diseases, macrophomina, causes the plants to die, and the field workers have to go through the entire field and detect the faulty plants and replace them. By using drone RGB imaging from an altitude of 20m above the growing plots, gathering images and processing to produce an Orthomosaic and then uploading them to ATLAS analysis software tool, an agronomist was able to construct a detector of the faulty plants and use it for the entire plot. ATLAS detected the defective plants and produced 2 fold output :
- Count and show the defective plants on the Orthomosaic map.
- Coverage estimate of the defective plants in relation to the entire area.
The preparation and flight were about one hour and ATLAS analysis took about 30 min.
Images/ Detection results built in ATLAS: Plot 2 Ha.


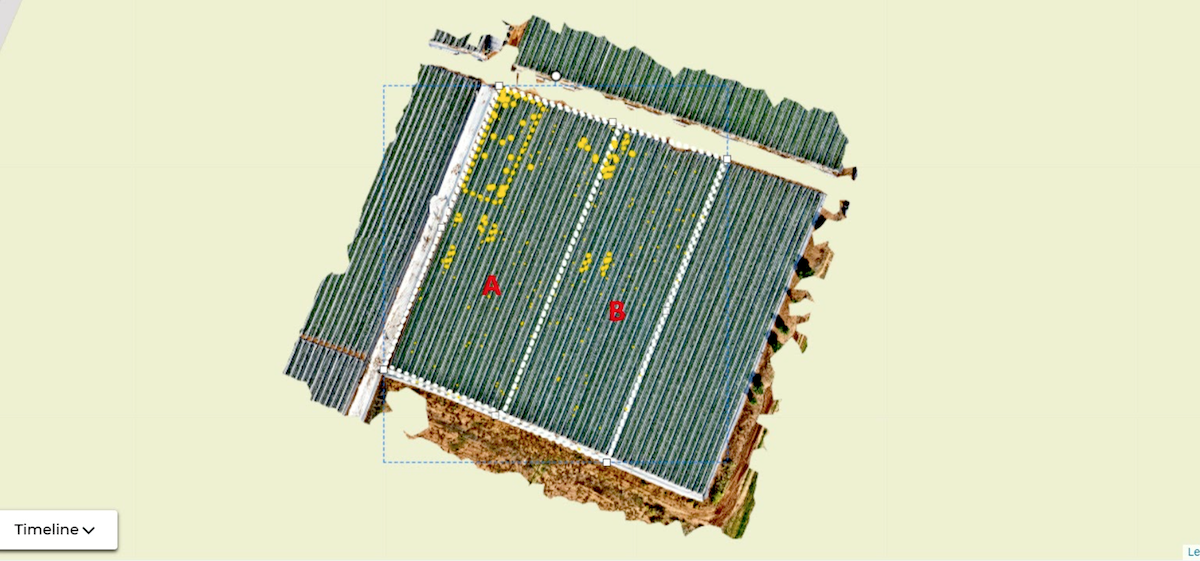
#melons
Growing water melons in open fields, is prone to weeds, such as: Prosopis farcta, which may be severe to the crops.
A flight with RGB Camera over the field produces a set of images, which were processed in ATLAS and then using the Detector to closely examine and allocate the invasive plants, thus using the Training feature of ATLAS to detect the training area, followed by detecting the entire field. 2-3 interactions were required in order to reach a high percentage of detection.
David Behr, CEO at Agro-Drone comments : “Using ATLAS gave an agronomist a fast and accurate insight into the invasive species not having to scout the entire field, only reach the problematic areas”.
Customer: Agro-Drone (Israel)
Industry: Agriculture, Drone inspection, Data processing
Process:
- DJI M2P with RGB Camera
- Map storage and processing in ATLAS:
- Manual annotation: approx. 20-25 minutes.
- Initial machine learning detector training in ATLAS: approx. 35 minutes.
Result:
ATLAS produces a report for agronomists/growers. It avoids going through the entire field, by going only to the infested places. In addition, the area coverage is depicted by ATLAS.